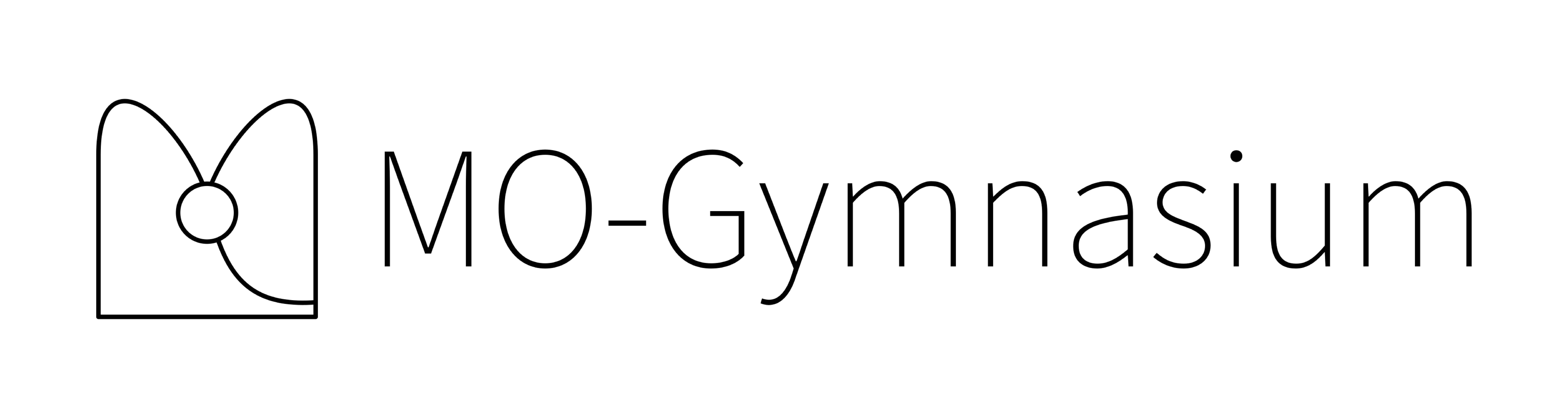
MO-Gymnasium is a standardized API and a suite of environments for multi-objective reinforcement learning (MORL)
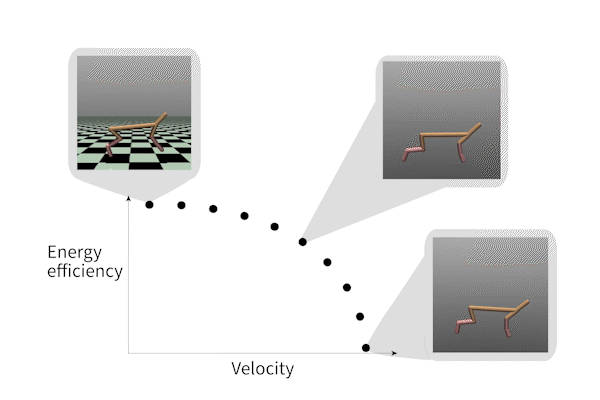
MO-Gymnasium is an open source Python library for developing and comparing multi-objective reinforcement learning algorithms by providing a standard API to communicate between learning algorithms and environments, as well as a standard set of environments compliant with that API. Essentially, the environments follow the standard Gymnasium API, but return vectorized rewards as numpy arrays.
The documentation website is at mo-gymnasium.farama.org, and we have a public discord server (which we also use to coordinate development work) that you can join here: https://discord.gg/bnJ6kubTg6.
API#
As for Gymnasium, the MO-Gymnasium API models environments as simple Python env
classes. Creating environment instances and interacting with them is very simple - here’s an example using the “minecart-v0” environment:
import gymnasium as gym
import mo_gymnasium as mo_gym
import numpy as np
# It follows the original Gymnasium API ...
env = mo_gym.make('minecart-v0')
obs, info = env.reset()
# but vector_reward is a numpy array!
next_obs, vector_reward, terminated, truncated, info = env.step(your_agent.act(obs))
# Optionally, you can scalarize the reward function with the LinearReward wrapper
env = mo_gym.LinearReward(env, weight=np.array([0.8, 0.2, 0.2]))
For details on multi-objective MDP’s (MOMDP’s) and other MORL definitions, see A practical guide to multi-objective reinforcement learning and planning.
Install#
To install MO-Gymnasium, use:
pip install mo-gymnasium
This does not include dependencies for all families of environments (some can be problematic to install on certain systems). You can install these dependencies for one family like pip install "mo-gymnasium[mujoco]"
or use pip install "mo-gymnasium[all]"
to install all dependencies.
Citing#
If you use this repository in your research, please cite:
@inproceedings{felten_toolkit_2023,
author = {Felten, Florian and Alegre, Lucas N. and Now{\'e}, Ann and Bazzan, Ana L. C. and Talbi, El Ghazali and Danoy, Gr{\'e}goire and Silva, Bruno C. {\relax da}},
title = {A Toolkit for Reliable Benchmarking and Research in Multi-Objective Reinforcement Learning},
booktitle = {Proceedings of the 37th Conference on Neural Information Processing Systems ({NeurIPS} 2023)},
year = {2023}
}